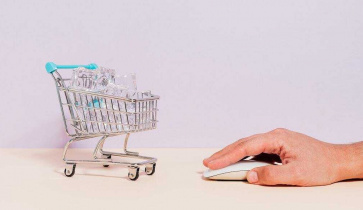
Ecommerce and Retail • Neto
Online credit card fraud continues to be a headache for Australian businesses, with an annual increase of 4.8% recorded in June last year1. From the various types of known credit card fraud, card-not-present transactions—the type used for ecommerce—now make up a colossal 85%1, making the problem more urgent than ever. Learning how to stop chargebacks through credit card fraud detection is essential to protect your business.
Thankfully, modern technology is coming to the rescue, in the guise of machine learning (ML, a function of Artificial Intelligence). For some, this might conjure up terrifying visions of pistol-wielding robots with crimson pupils, but in reality, machine learning is just an incredibly efficient method for creating predictive models from large amounts of data.
Payment gateways such as eWay, PayPal and Stripe are using machine learning techniques effectively to reduce credit card fraud, using a range of methods.
In theory, the more data that is made available for machine learning software, the greater the accuracy of its prediction models. A company with access to the data for billions of credit card transactions can use machine learning to quickly distinguish common identifiers for fraud, and use that information to create algorithms which automatically detect future fraudulent transactions. Over time, as more data is collected, the algorithm can be tweaked to become increasingly efficient—a self-learning, artificially intelligent system.
Facebook uses artificial intelligence to allow powerful targeted advertising, utilising its mammoth collection of data to predict the future actions of its users. Google uses AI to create software that can identify images in the real world, and is using this in combination with a satellite shipping tracking system to identify illegal fishers2, helping to keep delicate marine ecosystems in balance. With enough data, machine learning can be utilised to create powerful software.
With its free fraud detection software Radar, Stripe is leading the fray in the battle against credit card fraud, by utilising data from millions of global companies, processing billions of transactions. The company’s broad reach gives it unparalleled access to transactional credit card data, boasting an 89% chance of having witnessed a credit card on its network. This wealth of data allows them to create powerful fraud detection models, highly effective at protecting your business. Stripe is free to install with Neto, as part of our payment features.
One of the biggest problems to arise from fraudulent behaviour is the existence of false positives—flagging a transaction as fraudulent, when it’s actually a real customer, who may leave your online store in a huff because you’ve rejected their credit card.
Machine learning reduces false positives by considering multiple aspects of the transaction3: the sale amount; how long the transaction takes; how many different cards have been used; where the user is located, and many many more. By analysing multiple behavioural patterns, the system is able to predict fraud more effectively, choosing to either block the transaction completely, or ask for additional security information using a globally-supported service such as Dynamic 3D Secure. As more data is accumulated over time, the software is able to form increasingly adept fraud detection techniques, with high levels of confidence, teaching itself the best practices of ecommerce fraud prevention.
One of the ways that typical fraud detection software identifies fraud is by analysing manually flagged transactions for a business, and then building algorithms based on the data. For example, if a single customer tries to use multiple cards in short bursts, this may be identified as potentially fraudulent.
Fraud patterns change over time, so to become effective, the software may require fraudulent transactions to continue being flagged by somebody within the business, for an indefinite period. This can be incredibly time-consuming, and there’s also a chance that the person doing the manual flagging gets it wrong.
If software has access to enough historical and current data, it can learn to spot fraud classifiers all by itself, making manual flagging redundant. This could end up saving a business a lot of wasted effort, freeing up time for more valuable activities. With well-designed credit card fraud prevention software, your ecommerce platform becomes more secure.
Online tricksters use proxies to hide their true location, pretending to be in another location, using another computer. Stripe has developed a way to detect potential proxy users, by comparing the time it takes to connect with the user’s IP address (their unique location) with the time it takes to connect with their browser. A large discrepancy between the two is indicative of a proxy being used, which is considered a red flag by Stripe.
**
As online sales continue to increase, so will the likelihood of fraud. Modern problems require modern solutions, and machine learning is a potent weapon in the battle against online credit card fraud. As fraud-fighting artificial intelligence systems continue to self-improve, and are increasingly enhanced by their owners, we may begin to see a reduction in online credit card fraud.
References
Article photo by Franki Chamaki on Unsplash